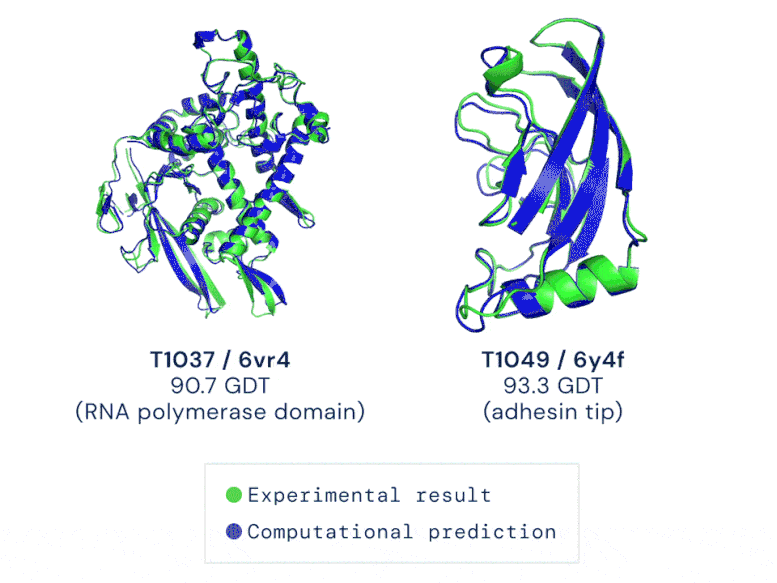
Two examples of protein targets in the free modeling category. AlphaFold predicts highly accurate structures measured against the experimental result. Credit: DeepMind
DeepMind develops AI solution to 50-year-old protein challenge, creating potential to accelerate biological research.
In a major scientific advance, the latest version of DeepMind’s AI system AlphaFold has been recognized as a solution to the 50-year-old grand challenge of protein structure prediction, often referred to as the ‘protein folding problem’, according to a rigorous independent assessment. This breakthrough could significantly accelerate biological research over the long term, unlocking new possibilities in disease understanding and drug discovery among other fields.
Today, results from CASP14 show that DeepMind’s latest AlphaFold system achieves unparalleled levels of accuracy in structure prediction. The system is able to determine highly-accurate structures in a matter of days. CASP, the Critical Assessment of protein Structure Prediction, is a biennial community-run assessment started in 1994, and the gold standard for assessing predictive techniques. Participants must blindly predict the structure of proteins that have only recently — or in some cases not yet — been experimentally determined, and wait for their predictions to be compared to experimental data.
CASP uses the “Global Distance Test (GDT)” metric to assess accuracy, ranging from 0-100. The new AlphaFold system achieves a median score of 92.4 GDT overall across all targets. The system’s average error is approximately 1.6 Angstroms — about the width of an atom. According to Professor John Moult, Co-founder and Chair of CASP, a score of around 90 GDT is informally considered to be competitive with results obtained from experimental methods.
Professor John Moult, Co-Founder and Chair of CASP, University of Maryland said:
“We have been stuck on this one problem — how do proteins fold up — for nearly 50 years. To see DeepMind produce a solution for this, having worked personally on this problem for so long and after so many stops and starts wondering if we’d ever get there, is a very special moment.”
Why protein structure prediction matters
Proteins are essential to life and their shapes are closely linked with their functions. The ability to predict protein structures accurately enables a better understanding of what they do and how they work. There are currently over 200 million proteins in the main database and only a fraction of their 3D structures have been mapped out.
A major challenge is the astronomical number of ways a protein could theoretically fold before settling into its final 3D structure. Many of the greatest challenges facing society, like developing treatments for diseases or finding enzymes that break down industrial waste, are fundamentally tied to proteins and the role they play. Determining protein shapes and functions is a major field of scientific research, primarily using experimental techniques that can take years of painstaking and laborious work per structure, and require the use of multi-million dollar specialized equipment.
DeepMind’s approach to the protein folding problem
This breakthrough builds on DeepMind’s first entry at CASP13 in 2018, where the initial version of AlphaFold achieved the highest level of accuracy among all participants. Now, DeepMind has developed new deep learning architectures for CASP14, drawing inspiration from the fields of biology, physics, and machine learning, as well as the work of many scientists in the protein folding field over the past half-century.
A folded protein can be thought of as a “spatial graph”, where residues are the nodes and edges connect the residues in close proximity. This graph is important for understanding the physical interactions within proteins, as well as their evolutionary history. For the latest version of AlphaFold used at CASP14, DeepMind created an attention-based neural network system, trained end-to-end, that attempts to interpret the structure of this graph, while reasoning over the implicit graph that it’s building. It uses evolutionarily related sequences, multiple sequence alignment (MSA), and a representation of amino acid residue pairs to refine this graph.
By iterating this process, the system develops strong predictions of the underlying physical structure of the protein. Additionally, AlphaFold can predict which parts of each predicted protein structure are reliable using an internal confidence measure.
The system was trained on publicly available data consisting of ~170,000 protein structures from the protein data bank, using a relatively modest amount of computing by modern machine learning standards — approximately 128 TPUv3-cores (roughly equivalent to ~100-200 GPUs) run over a few weeks.
Potential for real world impact
DeepMind is excited to collaborate with others to learn more about AlphaFold’s potential, and the AlphaFold team is looking into how protein structure predictions could contribute to the understanding of certain diseases with a few specialist groups.
There are also signs that protein structure prediction could be useful in future pandemic response efforts, as one of many tools developed by the scientific community. Earlier this year, DeepMind predicted several protein structures of the SARS-CoV-2 virus, and impressively quick work by experimentalists has now confirmed that AlphaFold achieved a high degree of accuracy on its predictions.
AlphaFold is one of DeepMind’s most significant advances to date. But as with all scientific research, there’s still much to be done, including figuring out how multiple proteins form complexes, how they interact with DNA, RNA, or small molecules, and how to determine the precise location of all amino acid side chains.
As with its earlier CASP13 AlphaFold system, DeepMind is planning to submit a paper detailing the workings of this system to a peer-reviewed journal in due course, and is simultaneously exploring how best to provide broader access to the system in a scalable way.
AlphaFold breaks new ground in demonstrating the stunning potential for AI as a tool to aid fundamental scientific discovery. DeepMind looks forward to collaborating with others to unlock that potential.
Statements from independent scientists:
Professor Venki Ramakrishnan, Nobel Laureate and President of the Royal Society
“This computational work represents a stunning advance on the protein-folding problem, a 50-year-old grand challenge in biology. It has occurred decades before many people in the field would have predicted. It will be exciting to see the many ways in which it will fundamentally change biological research.”
Professor Dame Janet Thornton, Director Emeritus & Senior Scientist, EMBL-EBI
“What the DeepMind team has managed to achieve is fantastic and will change the future of structural biology and protein research. After decades of studying proteins, the molecules that provide the structure and functions of all living things, I awoke this morning feeling that progress has been made.”
Arthur D. Levinson, PhD, Founder & CEO Calico, Former Chairman & CEO, Genentech
“AlphaFold is a once in a generation advance, predicting protein structures with incredible speed and precision. This leap forward demonstrates how computational methods are poised to transform research in biology and hold much promise for accelerating the drug discovery process.”
Professor Andrei Lupas, Director, Max Planck Institute for Developmental Biology
“AlphaFold’s astonishingly accurate models have allowed us to solve a protein structure we were stuck on for close to a decade, relaunching our effort to understand how signals are transmitted across cell membranes.”
Professor Ewan Birney, Deputy Director General EMBL, Director EMBL-EBI
“I nearly fell off my chair when I saw these results. I know how rigorous CASP is — it basically ensures that computational modeling must perform on the challenging task of ab initio protein folding. It was humbling to see that these models could do that so accurately. There will be many aspects to understand but this is a huge advance for science.”
Statements from DeepMind / Alphabet:
Demis Hassabis, PhD, Founder and CEO, DeepMind
“The ultimate vision behind DeepMind has always been to build AI and then use it to help further our knowledge about the world around us by accelerating the pace of scientific discovery. For us, AlphaFold represents the first proof point for that thesis. This advance is our first major breakthrough in a long-standing grand challenge in science, which we hope will have a big real-world impact on disease understanding and drug discovery.”
Pushmeet Kohli, PhD, Head of AI for Science, DeepMind
“These incredible results are testament to DeepMind’s unique research philosophy — bringing together mission-focused, multidisciplinary teams to target ambitious scientific goals. Critical assessments like CASP are important for driving research progress, and we look forward to building on this work, deepening our understanding of proteins and biological mechanisms, and opening up new avenues of exploration.”
John Jumper, PhD, AlphaFold Lead, DeepMind
“Protein biology is fantastically complex and defies simple characterization. Our team’s work demonstrates that machine learning techniques are finally able to meet the complexity of describing these incredible protein machines, and we are truly excited to see what new breakthroughs in both human health and fundamental biology it will bring.”
Kathryn Tunyasuvunakool, PhD, Science Engineer, DeepMind
“The ability to predict high accuracy protein structures with AI could change how we approach biology, with potential applications in drug design and bioremediation. Particularly for experimentally challenging proteins, good predictive techniques could make a huge difference.”
Sundar Pichai, CEO, Google and Alphabet
“This is an incredible AI-powered breakthrough in protein folding, which will help us better understand one of life’s most fundamental building blocks. This huge leap forward from DeepMind has immediate practical implications, enabling researchers to tackle new and difficult problems, from future pandemic response to environmental sustainability.”
Read DeepMind AI Solution to a 50-Year-Old Science Challenge Could “Revolutionize Medical Research” for more on this topic.
About DeepMind
DeepMind is a multidisciplinary team of scientists, engineers, machine learning experts and more, working together to research and build safe AI systems that learn how to solve problems and advance scientific discovery for all.
Best-known for developing AlphaGo, the first program to beat a world champion at the complex game of Go, DeepMind has published over 1000 research papers — including more than a dozen in Nature and Science — and achieved breakthrough results in many challenging AI domains from StarCraft II to protein folding.
DeepMind was founded in London in 2010, and joined forces with Google in 2014 to accelerate its work. Since then, its community has expanded to include teams in Alberta, Montreal, Paris, and Mountain View in California.
Another astounding breakthrough for artificial intelligence and the discipline is still in its infancy. How can anyone deny that AI will soon exceed human capability in every endeavor? The delicious irony is that the future of the human race lies not in our biology, but in our machines.
Here it explicitly exceed our innate capabilities (though it is also a nice demonstration of applying them).
I doubt “maximization” is generally possible – certainly evolution never attains such moving targets – but I have no doubt that the “singularity” is a futurist fantasy from someone that sell books and little else. I don’t think there is any science on it (but I could be mistaken).
Oops. Sorry, the last paragraph was intended as response to someone else – please dismiss.
While AlphaFold is giving amazing results, it ought to be viewed as a paradigm shift, not a specific problem solver. This breakthrough could as easily have been in many different fields, from football to adult gene therapy improvements. The Singularity is coming – our culture is holding back technological advancement, and when AI takes the lead it bypasses obsolete and inefficient human societal roadblocks and hangups to maximize favorable results.
I doubt “maximization” is generally possible – certainly evolution never attains such moving targets – but I have no doubt that the “singularity” is a futurist fantasy from someone that sell books and little else. I don’t think there is any science on it (but I could be mistaken).
> edges connect the residues in close proximity
“Close proximity” is a redundancy.
Are you sure?! Doesn’t that refer to physical proximity in the proteins as mapped to the amino acids sequence as edges in the graph? The primary protein structure may be seen as redundant if you organize the graph thusly, but the secondary strand and sheets and tertiary fold motifs shouldn’t be. [I think AlphaFold doesn’t do the quaternary structure level of aggregating subunits into multimers yet, I read that it is in the plan though.]
Cool – testing evolution and the use of machine learning for “accelerating the pace of scientific discovery” all at once!
We have to give a Chance to Nature also in determining protein structure. We have a variety of mice, rats, hamsters and guineapigs. Take a pure protein solution and put it into their Abdominal cavities. After 1 month, collect it and see whether there has been a change in its sructure and later on why it happened.