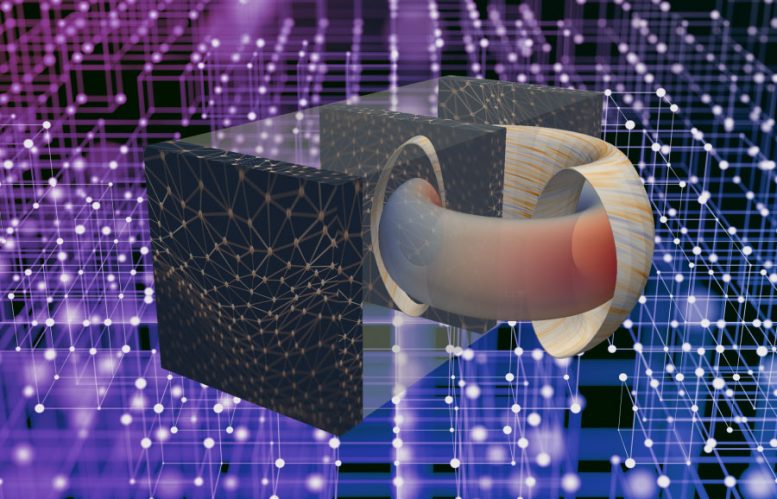
Depiction of fusion research on a doughnut-shaped tokamak enhanced by artificial intelligence. (Depiction by Eliot Feibush/PPPL and Julian Kates-Harbeck/Harvard University)
Artificial intelligence (AI), a branch of computer science that is transforming scientific inquiry and industry, could now speed the development of safe, clean and virtually limitless fusion energy for generating electricity. A major step in this direction is under way at the U.S. Department of Energy’s (DOE) Princeton Plasma Physics Laboratory (PPPL) and Princeton University, where a team of scientists working with a Harvard graduate student is for the first time applying deep learning — a powerful new version of the machine learning form of AI — to forecast sudden disruptions that can halt fusion reactions and damage the doughnut-shaped tokamaks that house the reactions.
Promising new chapter in fusion research
“This research opens a promising new chapter in the effort to bring unlimited energy to Earth,” Steve Cowley, director of PPPL, said of the findings, which are reported in the current issue of Nature magazine. “Artificial intelligence is exploding across the sciences and now it’s beginning to contribute to the worldwide quest for fusion power.”
Fusion, which drives the sun and stars, is the fusing of light elements in the form of plasma — the hot, charged state of matter composed of free electrons and atomic nuclei — that generates energy. Scientists are seeking to replicate fusion on Earth for an abundant supply of power for the production of electricity.
Crucial to demonstrating the ability of deep learning to forecast disruptions — the sudden loss of confinement of plasma particles and energy — has been access to huge databases provided by two major fusion facilities: the DIII-D National Fusion Facility that General Atomics operates for the DOE in California, the largest facility in the United States, and the Joint European Torus (JET) in the United Kingdom, the largest facility in the world, which is managed by EUROfusion, the European Consortium for the Development of Fusion Energy. Support from scientists at JET and DIII-D has been essential for this work.
The vast databases have enabled reliable predictions of disruptions on tokamaks other than those on which the system was trained — in this case from the smaller DIII-D to the larger JET. The achievement bodes well for the prediction of disruptions on ITER, a far larger and more powerful tokamak that will have to apply capabilities learned on today’s fusion facilities.
The deep learning code, called the Fusion Recurrent Neural Network (FRNN), also opens possible pathways for controlling as well as predicting disruptions.
Most intriguing area of scientific growth
“Artificial intelligence is the most intriguing area of scientific growth right now, and to marry it to fusion science is very exciting,” said Bill Tang, a principal research physicist at PPPL, coauthor of the paper and lecturer with the rank and title of professor in the Princeton University Department of Astrophysical Sciences who supervises the AI project. “We’ve accelerated the ability to predict with high accuracy the most dangerous challenge to clean fusion energy.”
Unlike traditional software, which carries out prescribed instructions, deep learning learns from its mistakes. Accomplishing this seeming magic are neural networks, layers of interconnected nodes — mathematical algorithms — that are “parameterized,” or weighted by the program to shape the desired output. For any given input the nodes seek to produce a specified output, such as correct identification of a face or accurate forecasts of a disruption. Training kicks in when a node fails to achieve this task: the weights automatically adjust themselves for fresh data until the correct output is obtained.
A key feature of deep learning is its ability to capture high-dimensional rather than one-dimensional data. For example, while non-deep learning software might consider the temperature of a plasma at a single point in time, the FRNN considers profiles of the temperature developing in time and space. “The ability of deep learning methods to learn from such complex data make them an ideal candidate for the task of disruption prediction,” said collaborator Julian Kates-Harbeck, a physics graduate student at Harvard University and a DOE-Office of Science Computational Science Graduate Fellow who was lead author of the Nature paper and chief architect of the code.
Training and running neural networks rely on graphics processing units (GPUs), computer chips first designed to render 3D images. Such chips are ideally suited for running deep learning applications and are widely used by companies to produce AI capabilities such as understanding spoken language and observing road conditions by self-driving cars.
Kates-Harbeck trained the FRNN code on more than two terabytes (1012) of data collected from JET and DIII-D. After running the software on Princeton University’s Tiger cluster of modern GPUs, the team placed it on Titan, a supercomputer at the Oak Ridge Leadership Computing Facility, a DOE Office of Science User Facility, and other high-performance machines.
A demanding task
Distributing the network across many computers was a demanding task. “Training deep neural networks is a computationally intensive problem that requires the engagement of high-performance computing clusters,” said Alexey Svyatkovskiy, a coauthor of the Nature paper who helped convert the algorithms into a production code and now is at Microsoft. “We put a copy of our entire neural network across many processors to achieve highly efficient parallel processing,” he said.
The software further demonstrated its ability to predict true disruptions within the 30-millisecond time frame that ITER will require, while reducing the number of false alarms. The code now is closing in on the ITER requirement of 95 percent correct predictions with fewer than 3 percent false alarms. While the researchers say that only live experimental operation can demonstrate the merits of any predictive method, their paper notes that the large archival databases used in the predictions, “cover a wide range of operational scenarios and thus provide significant evidence as to the relative strengths of the methods considered in this paper.”
From prediction to control
The next step will be to move from prediction to the control of disruptions. “Rather than predicting disruptions at the last moment and then mitigating them, we would ideally use future deep learning models to gently steer the plasma away from regions of instability with the goal of avoiding most disruptions in the first place,” Kates-Harbeck said. Highlighting this next step is Michael Zarnstorff, who recently moved from deputy director for research at PPPL to chief science officer for the laboratory. “Control will be essential for post-ITER tokamaks – in which disruption avoidance will be an essential requirement,” Zarnstorff noted.
Progressing from AI-enabled accurate predictions to realistic plasma control will require more than one discipline. “We will combine deep learning with basic, first-principle physics on high-performance computers to zero in on realistic control mechanisms in burning plasmas,” said Tang. “By control, one means knowing which ‘knobs to turn’ on a tokamak to change conditions to prevent disruptions. That’s in our sights and it’s where we are heading.”
Support for this work comes from the Department of Energy Computational Science Graduate Fellowship Program of the DOE Office of Science and National Nuclear Security Administration; from Princeton University’s Institute for Computational Science and Engineering (PICsiE); and from Laboratory Directed Research and Development funds that PPPL provides. The authors wish to acknowledge assistance with high-performance supercomputing from Bill Wichser and Curt Hillegas at PICSciE; Jack Wells at the Oak Ridge Leadership Computing Facility; Satoshi Matsuoka and Rio Yokata at the Tokyo Institute of Technology; and Tom Gibbs at NVIDIA Corp.
Reference: “Real-time capable modeling of neutral beam injection on NSTX-U using neural networks” by M.D. Boyer, S. Kaye and K. Erickson, 22 March 2019, Nuclear Fusion.
DOI: 10.1088/1741-4326/ab0762
I have been following the development of this technology and the the sudden advancements are totally mind boggling. I am ready for it to happen. This technology will transform our planet for the benefit of all people and promise a incredible future for my children, their children, and their children. I have been reading about this for decades and it looks like it is going to happen.
Hmmm….”steer plasma away from disruptions”. IF ONLY we keep repeating how close to solving fusion we are we can move into imaginary power in our world of the Emperor’s new clothes.
I’ve read that if we had pursued perfecting the internal combustion engine we could have by now had 50% instead of 10%
Corporates did not want to retool, its easier to claim ‘next big thing’ by substituting one slightly different application for one in use.
AI design and or control of hotfusion will move it from never to never with AI! Brillouin Energy is already beyond Thermal energy out more than twice the electrical energy in. Full 3rd party verification by SRI. Currently have multiple test system in operation with interchangeable parts. Performance always follows the catalyst rod across all reactors. Now raising money to finish manufacturing engineering. This will stabilize the catalyst rod production process for mass production and product role out by OEMs.
And just think about this… Rick Perry is in charge of the DOE.
Contrary to popular belief fussion energy isn’t the only way for endless energy, there is one smple and cheaper way that scientist and engineers around the world has been neglected for centuries. It does not need $25B to create a full productive prototype. A billion one is large enough for the project, I just don’t know how to raise enough fund for it.
We already have limitless free energy. It’s called renewable energy. Wind, water and solar energy…
Dog has eaten our homework on this project for too many decades. Enough build it already!
Hopefully one mistake by the A.I. computers doesn’t doom us in any way!
Can someone give me a brief summary of this article?
Doesn’t gravity always demand less from more?
B.S., fusion energy is NOT clean. AI will not be giving us any good fruit from a poison tree.
From the article:
“The neutron radiation damage in the solid vessel wall is expected to be worse than in fission reactors because of the higher neutron energies.”
“it’s truly bizarre that it would ever be hailed as the ideal electrical energy source. In fact, these neutron streams lead directly to four regrettable problems with nuclear energy: 1) radiation damage to structures; 2) radioactive waste; 3) the need for biological shielding; 4) and the potential for the production of weapons-grade plutonium 239—thus adding to the threat of nuclear weapons proliferation (not lessening it, as fusion proponents would have it.)”
Fusion reactors, along with fission reactors are a big fail to the environment and all life. The research needs to be scrapped, and the money allocated to cleaning up radioactive waste instead, transitioning us AWAY from centralized power sources, to DE-centralized and sustainable wind/solar.
https://thebulletin.org/2017/04/fusion-reactors-not-what-theyre-cracked-up-to-be/
“
Except that this kind of fusion reactor emulates/replicates what the sun is doing. Fusion isn’t always with radiactive elements, that’s where you are wrong. How can you get plutonium 239 from hydrogen? That’s just stupid. Please inform yourself instead of writing nonsense.