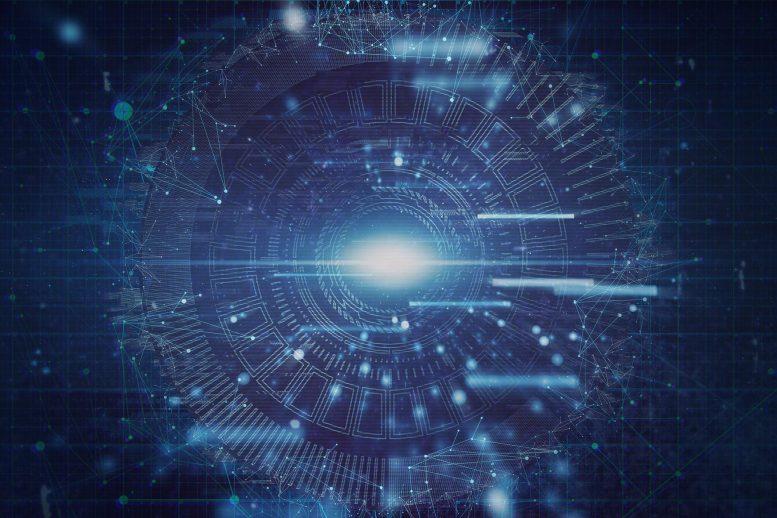
A new computer algorithm that predicts planetary orbits could be modified to improve control of plasma in fusion facilities.
A novel computer algorithm, or set of rules, that accurately predicts the orbits of planets in the solar system could be adapted to better predict and control the behavior of the plasma that fuels fusion facilities designed to harvest on Earth the fusion energy that powers the sun and stars.
The algorithm, devised by a scientist at the U.S. Department of Energy’s (DOE) Princeton Plasma Physics Laboratory (PPPL), applies machine learning, the form of artificial intelligence (AI) that learns from experience, to develop the predictions. “Usually in physics, you make observations, create a theory based on those observations, and then use that theory to predict new observations,” said PPPL physicist Hong Qin, author of a paper detailing the concept in Scientific Reports. “What I’m doing is replacing this process with a type of black box that can produce accurate predictions without using a traditional theory or law.”
Qin (pronounced Chin) created a computer program into which he fed data from past observations of the orbits of Mercury, Venus, Earth, Mars, Jupiter, and the dwarf planet Ceres. This program, along with an additional program known as a “serving algorithm,” then made accurate predictions of the orbits of other planets in the solar system without using Newton’s laws of motion and gravitation. “Essentially, I bypassed all the fundamental ingredients of physics. I go directly from data to data,” Qin said. “There is no law of physics in the middle.”
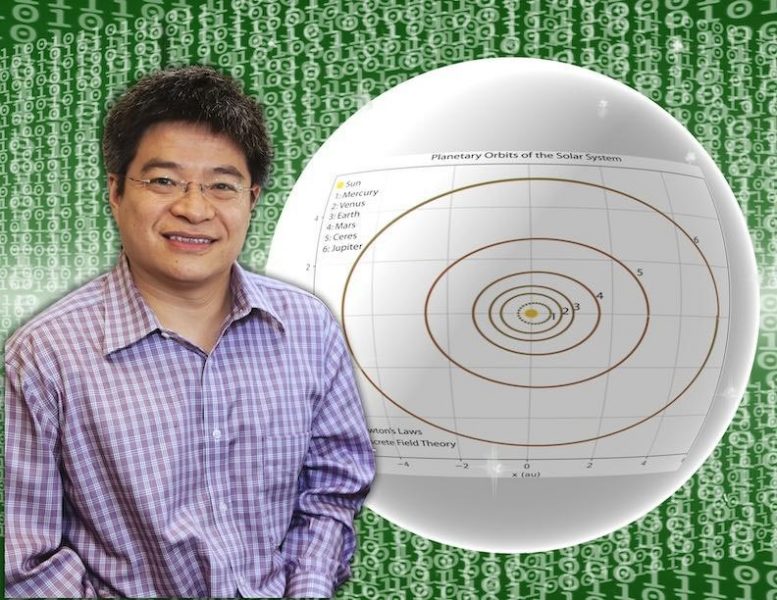
PPPL physicist Hong Qin in front of images of planetary orbits and computer code. Credit: Elle Starkman / PPPL Office of Communications
The program does not happen upon accurate predictions by accident. “Hong taught the program the underlying principle used by nature to determine the dynamics of any physical system,” said Joshua Burby, a physicist at the DOE’s Los Alamos National Laboratory who earned his Ph.D. at Princeton under Qin’s mentorship. “The payoff is that the network learns the laws of planetary motion after witnessing very few training examples. In other words, his code really ‘learns’ the laws of physics.”
Machine learning is what makes computer programs like Google Translate possible. Google Translate sifts through a vast amount of information to determine how frequently one word in one language has been translated into a word in another language. In this way, the program can make an accurate translation without actually learning either language.
The process also appears in philosophical thought experiments like John Searle’s Chinese Room. In that scenario, a person who did not know Chinese could nevertheless “translate” a Chinese sentence into English or any other language by using a set of instructions, or rules, that would substitute for understanding. The thought experiment raises questions about what, at root, it means to understand anything at all, and whether understanding implies that something else is happening in the mind besides following rules.
Qin was inspired in part by Oxford philosopher Nick Bostrom’s philosophical thought experiment that the universe is a computer simulation. If that were true, then fundamental physical laws should reveal that the universe consists of individual chunks of space-time, like pixels in a video game. “If we live in a simulation, our world has to be discrete,” Qin said. The black box technique Qin devised does not require that physicists believe the simulation conjecture literally, though it builds on this idea to create a program that makes accurate physical predictions.
The resulting pixelated view of the world, akin to what is portrayed in the movie The Matrix, is known as a discrete field theory, which views the universe as composed of individual bits and differs from the theories that people normally create. While scientists typically devise overarching concepts of how the physical world behaves, computers just assemble a collection of data points.
Qin and Eric Palmerduca, a graduate student in the Princeton University Program in Plasma Physics, are now developing ways to use discrete field theories to predict the behavior of particles of plasma in fusion experiments conducted by scientists around the world. The most widely used fusion facilities are doughnut-shaped tokamaks that confine the plasma in powerful magnetic fields.
Fusion, the power that drives the sun and stars, combines light elements in the form of plasma — the hot, charged state of matter composed of free electrons and atomic nuclei that represents 99% of the visible universe — to generate massive amounts of energy. Scientists are seeking to replicate fusion on Earth for a virtually inexhaustible supply of power to generate electricity.
“In a magnetic fusion device, the dynamics of plasmas are complex and multi-scale, and the effective governing laws or computational models for a particular physical process that we are interested in are not always clear,” Qin said. “In these scenarios, we can apply the machine learning technique that I developed to create a discrete field theory and then apply this discrete field theory to understand and predict new experimental observations.”
This process opens up questions about the nature of science itself. Don’t scientists want to develop physics theories that explain the world, instead of simply amassing data? Aren’t theories fundamental to physics and necessary to explain and understand phenomena?
“I would argue that the ultimate goal of any scientist is prediction,” Qin said. “You might not necessarily need a law. For example, if I can perfectly predict a planetary orbit, I don’t need to know Newton’s laws of gravitation and motion. You could argue that by doing so you would understand less than if you knew Newton’s laws. In a sense, that is correct. But from a practical point of view, making accurate predictions is not doing anything less.”
Machine learning could also open up possibilities for more research. “It significantly broadens the scope of problems that you can tackle because all you need to get going is data,” Palmerduca said.
The technique could also lead to the development of a traditional physical theory. “While in some sense this method precludes the need of such a theory, it can also be viewed as a path toward one,” Palmerduca said. “When you’re trying to deduce a theory, you’d like to have as much data at your disposal as possible. If you’re given some data, you can use machine learning to fill in gaps in that data or otherwise expand the data set.”
Reference: “Machine learning and serving of discrete field theories” by Hong Qin, 9 November 2020, Scientific Reports.
DOI: 10.1038/s41598-020-76301-0
… a very logical next step, but now I think about decoherence in a quantum world and ask my self is any interaction, which is inevitable at real conditions, just a measurement, after all…
@xABBAAA Of course no measurement is *just* a measurement. Every single one represents a collapse of coherence. We cannot observe nature without changing it. One of the benefits of ML in particular and AI in general is its ability to infer data without observing it directly. Hopefully what we see moving forward is a greater understanding of how fundamental physics behaves unobserved.
I actually can back his statement up tho I wrote out the alignment after it had taken place tho and wasn’t aware of it I was in prison when the alignment happened and I drew this like a few years ago then after that I found the actual alignment which is what caught my eye because I had drawn it I found the paper and ever planet was right except Uranus it was down off to the side a bit and the only reason it was off is because I ran out of paper room.
It’s just pretty much gathering enough information about something as you can and go based of the knowledge and stuff sitting infront of to come to some kinda rational conclusion. That’s like is light a wave or a particle well tech there both it all just depends on its surroundings and how bright the light is I mean you see black spots when it’s to bright and you see white it’s pitch black so lol but mainly it’s both untill an observer is added then it acts as either one or the other and that’s just depending how the observer sees it act at that time.im sure it’s possible for the observer to observe and come back to the light and observé particle one time then see it as a wave the next I’m sure can be included
It’s a wave and a particle at the same time it all depends on the observer and whats being observed and how there feeling at the time or your emotional state during the time.
When time and space warp waves and particles depending on the observer and whats being observed bends with it.
A particle is a source of matter that exsist at a single point, and wave exsist everywhere except at the points that create them
Energy always “flows” through regions of space and time.
Prediction without understanding: From a pragmatic point of view, I can’t argue with useful results. From an epistemological point of view, I like understanding. I think science is more than just making predictions.
So I have great amount of data that I would like a “machine learning Algorithm” to run though it, BUT I find you must be a Professor or something to get access to the programing…the first part of this paper is what I am referring to… My data is all of the Star data in the HIP catalogue…
Before Newton we could predict the planets but observed from earth the movements were complex and only applied to the planets we could observed.
Newton gave us an explanation which then simplified the calculations and formulae.
It applied to more than the planets and we have used those same ideas ever since (with some relativity updates from Einstein) to do more than predict the orbits.
We can now send spacecraft to distant planets. We can keep satellites in accurate, stable orbits.
That’s more than simple prediction.
Prediction can be important but it is inot an explanation and of itself doesn’t throw light on a range of other problems.
Science comes from the latin word for knowledge, technology the study of an art or craft, and applied science is a part of that …
There is a term for that for humans: Cognition. One does not have to have been taught Newton’s Law of Gravity to know that when you let go of the apple, it will fall to the ground. So based on previous data, i.e., where everything that you let go falls to the ground, then you can accurately predict that the next time you let go of something, it will fall to the ground.
… don’t give you self way to much credit, you are just humans, and the nature is just a nature…
… The God works in mysterious ways, but so does nature and humans, too…
There are inherent flaws here in understanding how machine learning works. It’s not like predictions are being made by ML without knowing the laws of motion. The laws themselves are being “learned” in the training phase of the model only to be applied in the prediction phase. The ML model DOES NOT use the training data for making predictions at all. It only relies on the “laws” learned from it to approximate an “answer” to a “question”. That is not to say that the approximations derived from such ML models are necessarily worse than the ones derived from solutions to equations for the laws based on established theories. They often are worse but if our understanding of the laws themselves is on the lacking side, the ML and DL approximations can make useful predictions wich would otherwise be impossible to make. Or would take much longer to make given that equations accurately depicting some complex laws of physics are analytically unsolvable with numerical approach being very computationally expensive. However, the fact remains that the model learns the rules (call them it’s own “laws”) by observing data and interpolating or extrapolating as required based on those rules and not at all based on data that it used to learn those rules.
Reductio ad absurdum: Isac Newton was a non-machine learning model that was trained on observed data and thus learned a set of rules exactly like ML models do. The trained model stored his learned “parameters” in his famous laws of motion which are a so-so approximation of nature. Useful for some applications, useless for others. No difference whatsoever in how ML works.
Point being, call it a black box if you don’t care about learning more about how it works but don’t make spectacular false headlines about simple math.
Its an overstatement to say AI learning and predicting physical phenomenon questions scientific theory over this type of machine essentially interpolation between data points to “learn” to make accurate predictions. Very much of an overstatement. A superb example is the Standard Model Of Particle Physics. Many people this this is a “theory”. Its not, its a mathematical model that simply (actually brilliantly) reproduces particle characteristics that have been observed and predicts future particles like the Higgs which was accurately described by the model in advance. But this is not a theory because it has no underlying principles that explain the predicted outcomes from the model. In essence it is a man-made AI type thing where many brilliant minds created a Mathematical model based on group theory, symmetry, and elaborate mathematical permutations that did indeed produce future verified results. So in this sense it is precisely similar to machine AI produced predictions discussed in the very good article. But there is no underlying theory to the Standard Model. Its explains absolutely nothing in terms of a core central theoretical framework. We know nothing more from the model why and electron for example is what it is, etc. This is quite a contrast to Newtonian physics and GR for example that give underlying cause and effect as well as make perfectly confirmable predictions. Using AI alone to understand phenonomon is quite dangerous because it will disconnect humans understanding from AI predictions that are confirmed but not at all understood by humans in any way but a black box outcome of the AI generated results. That’s exactly what the Standard Model does. Work is ongoing to find a core theory explaining the totality of the Standard Model through a core theory much like Newton’s and Einstein’s theories. Those are understandings; AI in the sense used here is not. AI can still be very, very useful and in addition to being accurate may lead to new theories that explain outcomes.
WE want to know why as well as what.
I remember my first polynomial curve fit too!
I believe the underlying communication here is that, with the advent of ML and AI, it won’t be super paramount that all the underlying theories are known ahead before predictions are made during science experiments in the near future.
Our theories and laws can be pretty much said to be ML models that achieve 99.999% accuracy on the validation data available at the time they were formulated.
As it can be seen that the Newton model achieved lower accuracy when used on data that differs entirely from the one it was trained on (small mass and very fast particles)
We should not forget that theories are formulated from continuous observations of measured data which is similar to what a ML model does durning the training process. A ML model can better learn the theories associated with a dataset and thus simplifies the process for scientists.
There was a young man who stood near the parthenon, observing and hearing what he could from the closely knotted group of senators… till the one who was the whom all were queztioning… said to the person keeping the young man back at a distant… if the young man wants to be let in let him. Do not prohibit the youthful mind for it has not yet become a repetitive memory afraid of exploring new ideas and other and better explanations for the way things are… so the young man was let in. He was called so critiques.
I believe ml is useful in learning from data compared to humans. It is able to easily learn compared to a human which will take time to formulate its own formula which is a good thing to leverage on. However, I believe the physics theory is still important in explaining why it is happening. The answer to this allows human to understand and maybe even apply it to solve other puzzles in other situations that ml cannot solve especially in rare unseen situations. It will be naive to take sides and disgard the other. I believe both physics and ml should be use together and as cross references.