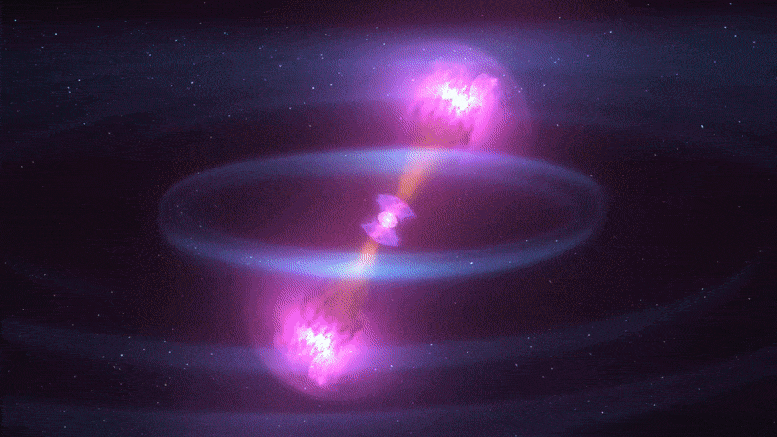
Two neutron stars colliding. Credit: NASA’s Goddard Space Flight Center/CI Lab
In the 2030s, gravitational-wave detectors will be thousands of times more sensitive than Advanced LIGO, Virgo, and KAGRA. The network of “third generation” (3G) observatories will almost certainly include Cosmic Explorer (US), Einstein Telescope (EU), and may include a Southern-hemisphere Cosmic-Explorer like observatory.
These amazing instruments will see every binary neutron star merger in the Universe, and most binary black holes out to redshifts beyond 10: hundreds of thousands, possibly millions, of resolvable signals per year. Many of these signals will be extremely loud, with signal-to-noise ratios in the thousands, facilitating breakthroughs in fundamental physics and cosmology.
And herein lies a challenge!
How do we extract all the information from these signals? On the surface it seems like a straightforward task: just keep on running parameter estimation like we’re already doing! But it turns out that our current parameter estimation methods don’t scale so well when signals are really loud, and very long in band.
To see why, we imagined a binary neutron star merger signal “GW370817,” which originated about 40 Mpc from Earth — roughly the distance of GW170817 (assuming 3G detectors are online in 2037, we’re guaranteed to observe a thousand or so binary neutron star mergers on August 17th, 2037!) A network of 3G detectors would observe GW370817 for 90 minutes, with a staggering signal-to-noise ratio of 2500. Analyzing this signal is around a thousand times more computationally expensive than analyzing a signal in today’s detectors — by our back-of-the-envelope estimates, it would take around 1000 years!
This prohibitive analysis time is a hurdle to astrophysics with 3G data, and it’s the problem we solve in our paper. To drive down the computation time, we developed “reduced order models” of gravitational-wave signals which allow us to infer binary neutron star properties using heavily compressed data, with almost no loss in accuracy. We reduced the computational cost of inference on 3G data by a factor of 13,000. Together with a pinch of parallel computing, we’re able to perform data analysis in a few hours. This is good news for astrophysics in the 3G era.
While the 2030s and 3G detectors are a few years away, our results and methods are useful for a wide range of theoretical and design studies, which are ramping up in lockstep with the development of the detector technology. For those old enough to remember, the first LISA mock-data challenges began in 2005, which gives a sense of how much exploratory work takes place before a detector is operational.
For the time being, there are plenty of interesting astrophysics questions we can start to think about in the context of 3G detectors: how well will we be able to measure the neutron star equation of state and the maximum mass of neutron stars? And what will this tell us about extreme matter? How well can neutron star spins be measured and can this tell us anything about supernova mechanisms? etc…Our results and method will facilitate this kind of theoretical work by enabling us to perform robust inferences on binary neutron star properties in mock 3G data.
Reference: “Bayesian inference for gravitational waves from binary neutron star mergers in third-generation observatories” by Rory Smith, Ssohrab Borhanian, Bangalore Sathyaprakash, Francisco Hernandez Vivanco, Scott Field, Paul Lasky, Ilya Mandel, Soichiro Morisaki, David Ottaway, Bram Slagmolen, Eric Thrane, Daniel Töyrä and Salvatore Vitale, 20 August 2021, Physical Review Letters.
DOI: 10.1103/PhysRevLett.127.081102
Be the first to comment on "Bayesian Inference for Gravitational Waves From Binary Neutron Star Mergers in 3G Observatories"