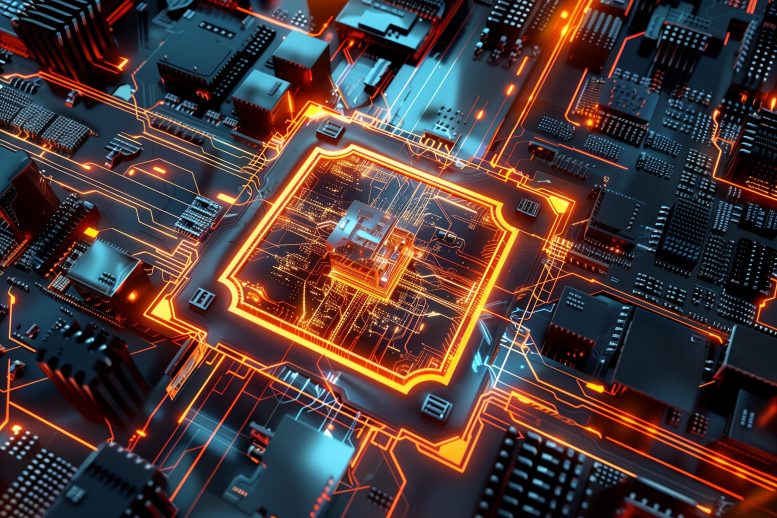
A new chip developed by Penn Engineers uses light to accelerate AI training, offering faster processing and reduced energy consumption while enhancing data privacy. (Artist’s concept.) Credit: SciTechDaily.com
An innovative new chip uses light for fast, efficient AI computations, promising a leap in processing speeds and privacy.
Penn Engineers have developed a new chip that uses light waves, rather than electricity, to perform the complex math essential to training AI. The chip has the potential to radically accelerate the processing speed of computers while also reducing their energy consumption.
The silicon-photonic (SiPh) chip’s design is the first to bring together Benjamin Franklin Medal Laureate and H. Nedwill Ramsey Professor Nader Engheta’s pioneering research in manipulating materials at the nanoscale to perform mathematical computations using light — the fastest possible means of communication — with the SiPh platform, which uses silicon, the cheap, abundant element used to mass-produce computer chips.
The interaction of light waves with matter represents one possible avenue for developing computers that supersede the limitations of today’s chips, which are essentially based on the same principles as chips from the earliest days of the computing revolution in the 1960s.
Collaborative Innovation in Chip Design
In a paper published today (February 15) in Nature Photonics, Engheta’s group, together with that of Firooz Aflatouni, Associate Professor in Electrical and Systems Engineering, describes the development of the new chip. “We decided to join forces,” says Engheta, leveraging the fact that Aflatouni’s research group has pioneered nanoscale silicon devices.
Their goal was to develop a platform for performing what is known as vector-matrix multiplication, a core mathematical operation in the development and function of neural networks, the computer architecture that powers today’s AI tools.
Instead of using a silicon wafer of uniform height, explains Engheta, “you make the silicon thinner, say 150 nanometers,” but only in specific regions. Those variations in height — without the addition of any other materials — provide a means of controlling the propagation of light through the chip, since the variations in height can be distributed to cause light to scatter in specific patterns, allowing the chip to perform mathematical calculations at the speed of light.
Advancements and Potential Applications
Due to the constraints imposed by the commercial foundry that produced the chips, Aflatouni says, this design is already ready for commercial applications, and could potentially be adapted for use in graphics processing units (GPUs), the demand for which has skyrocketed with the widespread interest in developing new AI systems. “They can adopt the Silicon Photonics platform as an add-on,” says Aflatouni, “and then you could speed up training and classification.”
In addition to faster speed and less energy consumption, Engheta and Aflatouni’s chip has privacy advantages: because many computations can happen simultaneously, there will be no need to store sensitive information in a computer’s working memory, rendering a future computer powered by such technology virtually unhackable. “No one can hack into a non-existing memory to access your information,” says Aflatouni.
Reference: “Inverse-designed low-index-contrast structures on a silicon photonics platform for vector–matrix multiplication” by Vahid Nikkhah, Ali Pirmoradi, Farshid Ashtiani, Brian Edwards, Firooz Aflatouni and Nader Engheta, 16 February 2024, Nature Photonics.
DOI: 10.1038/s41566-024-01394-2
This study was conducted at the University of Pennsylvania School of Engineering and Applied science and supported in part by a grant from the U.S. Air Force Office of Scientific Research’s (AFOSR) Multidisciplinary University Research Initiative (MURI) to Engheta (FA9550-21-1-0312) and a grant from the U.S. Office of Naval Research (ONR) to Afaltouni (N00014-19-1-2248).
Other co-authors include Vahid Nikkhah, Ali Pirmoradi, Farshid Ashtiani and Brian Edwards of Penn Engineering.
Be the first to comment on "At the Speed of Light: Unveiling the Chip That’s Reimagining AI Processing"