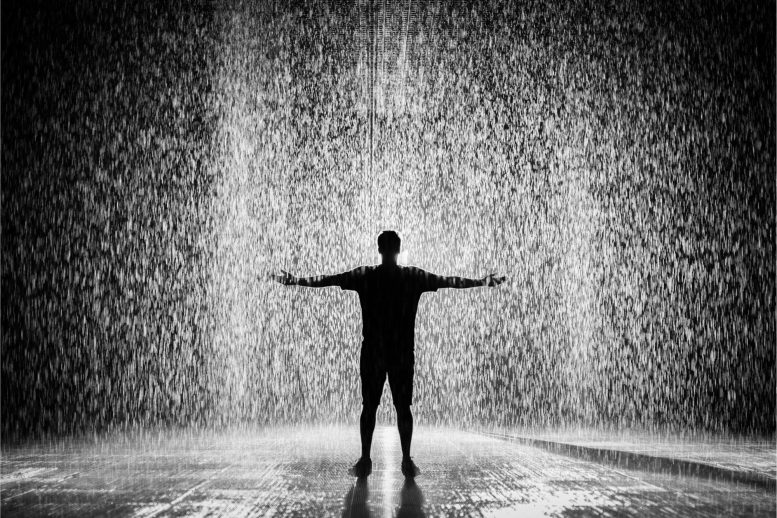
A recent study argues that scientists overly reliant on remote sensing and models miss important details about wet weather events, potentially affecting Earth systems models and scientific understanding. They advocate for direct, on-the-ground observations to improve data accuracy, inspire creativity, and enrich environmental education.
To be outstanding in one’s field, one may need to be out standing in one’s field.
An interdisciplinary research team led by John T. Van Stan from Cleveland State University argues that scientists should venture beyond the laboratory to directly observe weather phenomena like rain, snow, or occult deposition. In a paper published in the journal BioScience, the researchers contend that hands-on observation of storm events is crucial for comprehending the complexities of wet weather and its diverse impacts on the environment.
Recently, Van Stan and colleagues noted a trend in the scientific community towards relying on remote sensing to study storms and their consequences: “Natural scientists seem increasingly content to stay dry and rely on remote sensors and samplers, models, and virtual experiments to understand natural systems. Consequently, we can miss important stormy phenomena, imaginative inspirations, and opportunities to build intuition—all of which are critical to scientific progress.”
This type of “umbrella science,” they warn, can miss important localized events. For instance, in describing rainwater’s flow from the forest canopy to the soils, the authors note that “if several branches efficiently capture and drain stormwaters to the stem, rainwater inputs to near-stem soils can be more than 100 times greater.”
The authors also point out that important phenomena like low-lying fog events, vapor trapped beneath forest canopies, and condensate plumes may escape remote detection, yet be sensible to scientists on the ground. At the broader scale, these oversights can affect Earth systems models, which often underestimate canopy water storage. They argue that these errors may represent a “large potential bias in surface temperatures simulated by Earth systems models.”
Direct observation, however, has merits beyond remedying the shortcomings of “umbrella science.” Van Stan and colleagues see intrinsic value in firsthand storm experiences – not only for natural scientists, but also students studying climate change impacts on ecosystems. They claim that this immersive method enhances understanding, incites curiosity, and strengthens bonds with nature, thereby enriching environmental education, inspiring research, and preparing the future scientific community.
Reference: “Shower thoughts: why scientists should spend more time in the rain ” by John T Van Stan, Scott T Allen, Douglas P Aubrey, Z Carter Berry, Matthew Biddick, Miriam A M J Coenders-Gerrits, Paolo Giordani, Sybil G Gotsch, Ethan D Gutmann, Yakov Kuzyakov, Donát Magyar, Valentina S A Mella, Kevin E Mueller, Alexandra G Ponette-González, Philipp Porada, Carla E Rosenfeld, Jack Simmons, Kandikere R Sridhar, Aron Stubbins and Travis Swanson, 7 June 2023, BioScience.
DOI: 10.1093/biosci/biad044
The study was funded by the National Science Foundation, the Department of Agriculture and Fisheries, the McIntire Stennis Project, the DOE/US Department of Energy, and the U.S. Forest Service.
+1
There is far too much reliance on models today. Models can be helpful in understanding how dynamic systems work, but they are should not be trusted for predictions because they are difficult to verify and it is almost impossible to encompass all the variables that exist in the real world system. The only way that one can obtain the empirical data for verification is through field work. Models should be thought of as complex working hypotheses, not reality. The Scientific Method dictates that if the hypothesis is shown to be wrong, that it be should be replaced or modified. Without the empirical field data, one can’t know if the model is wrong.