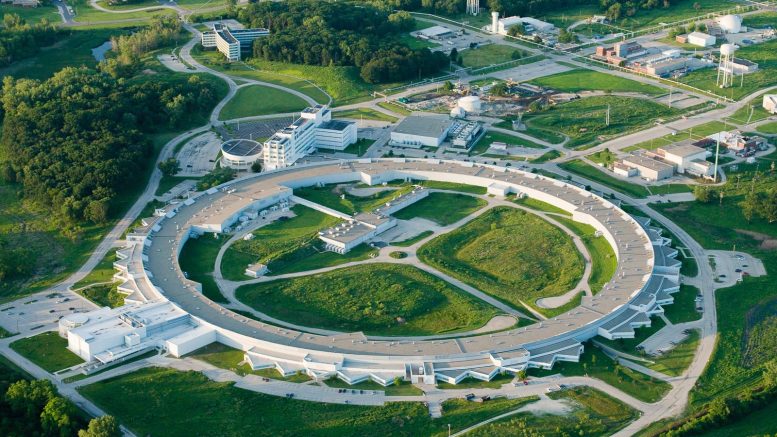
Researchers are using Argonne’s Advanced Photon Source, a massive kilometer-long accelerator, to analyze proteins that could lead to coronavirus treatments or vaccines. Credit: Argonne National Laboratory
Scientists at Argonne National Lab and University of Chicago search for COVID-19 treatments and analysis.
As COVID-19 makes its way around the world, scientists are working around the clock to analyze the virus to find new treatments and cures and predict how it will propagate through the population.
Some of their most powerful tools are supercomputers and particle accelerators, including those at Argonne National Laboratory, a U.S. Department of Energy laboratory affiliated with the University of Chicago.
X-rays for the cure
To make drugs that work against COVID-19, we first need to find a biochemical “key”—an inhibitor molecule that will nestle perfectly into the nooks and crannies of one or more of the 28 proteins that make up the virus. While researchers have already sequenced the genes of the virus, they also need to know what the shape of each protein looks like when it is fully assembled.
This requires a technique called macromolecular X-ray crystallography, in which scientists grow tiny crystals and then illuminate them in an incredibly high-energy X-ray beam to get a snapshot of its physical structure. Such X-ray beams exist only at a few specialized sites around the world, and one of them is Argonne’s Advanced Photon Source.
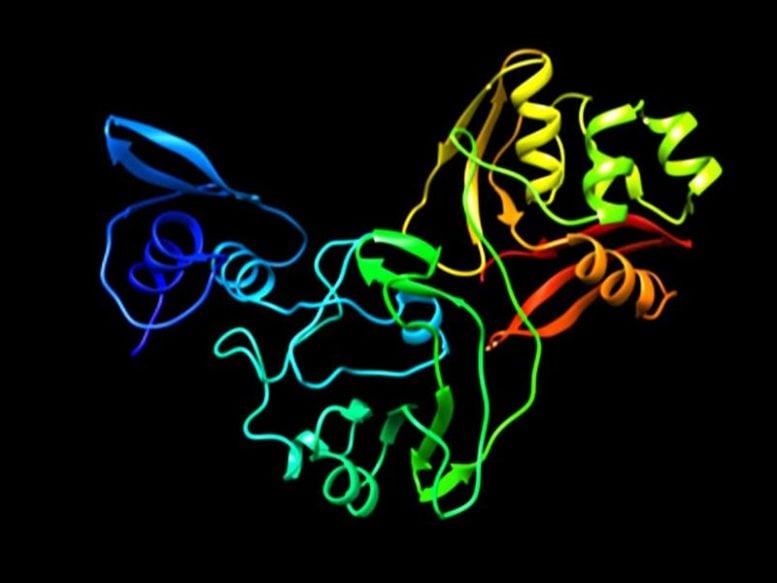
This newly mapped coronavirus protein, called Nsp15, helps the virus replicate. Credit: Joachimiak et al.
By mid-March, researchers from around the country had used the Advanced Photon Source to characterize roughly a dozen proteins from SARS-CoV-2. They even managed to catch glimpses of several of them with potential inhibitor molecules “in action.”
“The fortunate thing is that we have a bit of a head start,” said Bob Fischetti, who heads the Advanced Photon Source’s efforts in life sciences. “This virus is similar but not identical to the SARS outbreak in 2002, and 70 structures of proteins from several different coronaviruses had been acquired using data from APS beamlines prior to the recent outbreak.”
That means researchers have background information on how to express, purify and crystallize these proteins, which makes the structures come more quickly, “right now about a few a week,” he said.
Fischetti compared finding the right inhibitor for a protein to discovering a perfectly sized and shaped Lego brick that would snap perfectly into place. “These viral proteins are like big sticky balls—we call them globular proteins,” he said. “But they have pockets or crevices inside of them where inhibitors might bind.”
By using the X-rays, scientists can gain an atomic-level view of the recesses of a viral protein and see which possible inhibitors—either pre-existing or yet-to-be-developed—might reside best in the pockets of different proteins.
The difficulty with pre-existing inhibitors is that they tend to bind only weakly to COVID-19 proteins, which might mean extremely high doses that could cause complications in patients. According to Fischetti, the research teams are looking for an inhibitor that would have a much stronger affinity, enabling it to be administered as a drug that would have many fewer or no side effects.
Fischetti said the rapid pace of collaborative science with one common essential goal is unlike anything else he has seen in his career. “Everything is just moving so incredibly fast, and there are so many moving pieces that it’s hard to keep up with,” he said.
Computing the COVID-19 crisis
Supercomputers can play a role in searching for inhibitors, too. As part of the COVID-19 High Performance Computing Consortium, researchers at Argonne and the University of Chicago are joining forces with researchers from government, academia and industry in an effort that combines the power of 16 different supercomputing systems.
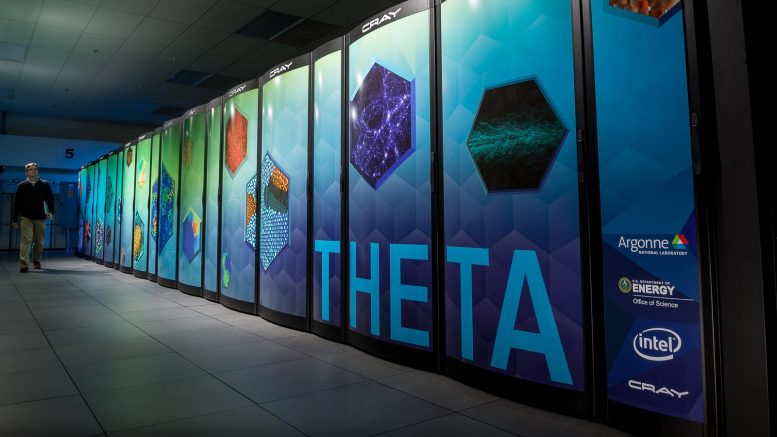
Supercomputers, such as Argonne’s Theta supercomputer, can whittle down the number of possible molecules for effective treatments. Credit: Argonne National Laboratory
At Argonne, researchers using the lab’s Theta supercomputer have linked up with other supercomputers from around the country. With their combined might, these supercomputers are powering simulations of how billions of different small molecules from drug libraries could interface and bind with different viral protein regions.
We already have databases of many potential drug candidates—such “libraries” include catalogs of small molecules that number in the hundreds of millions to billions. The problem, then, is how to narrow them down. Running individual simulations of each and every drug candidate for each viral protein, even with the supercomputers running 24/7, would take many years—a window of time that scientists don’t have.
Luckily, this is a problem tailor-made for new AI and machine learning techniques. To zero in on the most likely candidates as efficiently as possible, computational biologists can use these techniques to do a kind of educated filtration of possibilities.
“When we’re looking at this virus, we should be aware that it’s not likely just a single protein we’re dealing with—we need to look at all the viral proteins as a whole,” said Arvind Ramanathan, a computational biologist in Argonne’s Data Science and Learning division. “By using machine learning and artificial intelligence methods to screen for drugs across multiple target proteins in the virus, we may have a better pathway to an antiviral drug.”
“By using machine learning and artificial intelligence methods to screen for drugs across multiple target proteins in the virus, we may have a better pathway to an antiviral drug.” — Arvind Ramanathan, computational biologist at Argonne
Ten billion configurations are quickly whittled down to roughly six million positions that researchers can then do more intensive simulations on to see which would be the best candidates.
At the end of the day, they identify a handful of inhibitor candidates that can be fed back to scientists who can then actually make these molecules, inject them into viral proteins, and then use the Advanced Photon Source to check how well the molecules work. “It’s an iterative process,” said Rick Stevens, associate laboratory director of Argonne’s Computing, Environment, and Life Sciences directorate. “They feed structures to us, we feed our models to them—eventually we hope to find something that works well.”
Agents make the model
Computers can also help scientists simulate the spread of COVID-19 through the population. Argonne specializes in a kind of model called an “agent-based model.” Instead of just assuming a population of “average” people that do the same thing, agent-based models create a virtual crowd of people that act independently. The agent-based model that Argonne researchers have developed includes almost 3 million separate agents, each of whom can travel to any of 1.2 million different locations. The actions of each agent are determined by hourly schedules.
They are modifying this model to incorporate on-the-fly reports of the properties of the virus’s virulence that are being published every day in the scientific literature.
Currently, the Argonne team is developing a baseline simulation—in essence, to see what would happen to our communities if people carried on with business as usual. But the true goal is to be able to extensively model the various interventions—or possible additional interventions—that decision-makers can implement in order to slow the virus’s spread.
“Our models simulate individuals in a city interacting with each other,” said Argonne computational scientist Jonathan Ozik, who helps to lead Argonne’s epidemiological modeling research. “If there’s a school closure, we see people who are supposed to go to school not go to school, and we can look at population level outcomes, such as how the school closure affect how many people get exposed to the virus.”
The advantage of having a computer model of an entire city is that it represents a “laboratory” for decision-makers to see how different decisions might affect a population without actually having to implement them. “Knowing what decisions to make on a regional or national scale and when are crucial in this worldwide fight,” said Argonne scientist and pioneer in agent-based modeling Charles (Chick) Macal, who also leads the research. “We’re developing a model that will help give information about what decisions will be most effective.”
Funding for these efforts includes support from the National Institutes of Health and the U.S. Department of Energy, among many others.
Be the first to comment on "In the Fight Against Coronavirus, Supercomputers & Giant Accelerators Lend a Hand"