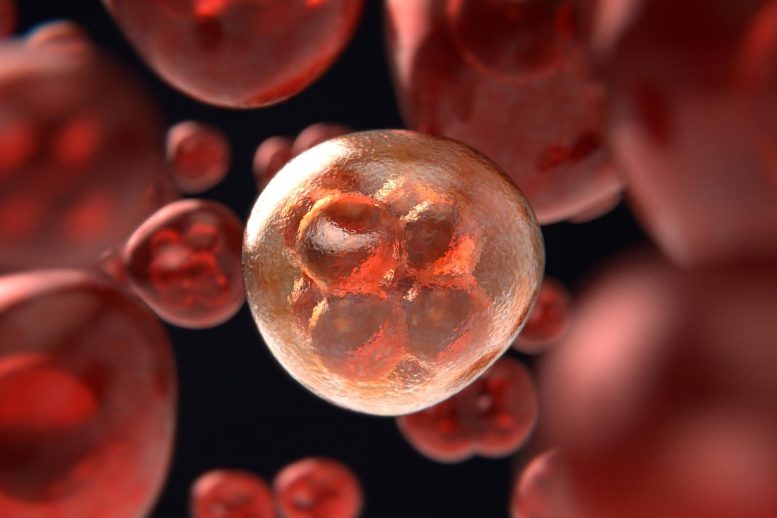
Cell identity refers to the unique characteristics and properties that distinguish one type of cell from another within an organism. This identity is determined by the expression of specific genes, which control the production of proteins that give cells their particular functions and structures.
Activation and expression of genes reveal similarities in cell patterns based on type and function throughout the tissues and organs. Understanding these patterns improves our comprehension of cells and offers insights into uncovering the underlying mechanisms of diseases.
The emergence of spatial transcriptomics technologies has enabled scientists to examine gene expression within the context of tissue samples as a whole. However, new computational techniques are necessary to process this information and facilitate the identification and comprehension of these gene expression patterns.
A research team led by Jian Ma, the Ray and Stephanie Lane Professor of Computational Biology in Carnegie Mellon University’s School of Computer Science, has developed a machine learning tool to fill this gap. Their paper on the method, called SPICEMIX, recently appeared as the cover story of Nature Genetics.
SPICEMIX helps researchers untangle the role different spatial patterns play in the overall gene expression of cells in complex tissues like the brain. It does so by representing each pattern with spatial metagenes — groups of genes that may be connected to a specific biological process and can display smooth or sporadic patterns across tissue.
The team, which included Ma; Benjamin Chidester, a project scientist in the Computational Biology Department; and Ph.D. students Tianming Zhou and Shahul Alam, used SPICEMIX to analyze spatial transcriptomics data from brain regions in mice and humans. They leveraged the unique capabilities of SPICEMIX to uncover the landscape of the brain’s cell types and spatial patterns.
“We were inspired by cooking when we chose the name,” Chidester said. “You can make all sorts of different flavors with the same set of spices. Cells may work in a similar way. They may use a common set of biological processes, but the specific combination they use gives them their unique identity.”
When applied to brain tissues, SPICEMIX identified spatial patterns of cell types in the brain more accurately than other methods. It also uncovered new expression patterns of brain cell types through the learned spatial metagenes.
“These findings may help us paint a more complete picture of the complexity of brain cell types,” Zhou said.
The number of studies using spatial transcriptomics technologies is growing rapidly, and SPICEMIX can help researchers make the most of this high-volume, high-dimensional data.
“Our method has the potential to advance spatial transcriptomics research and contribute to a deeper understanding of both basic biology and disease progression in complex tissues,” Ma said.
Reference: “SpiceMix enables integrative single-cell spatial modeling of cell identity” by Benjamin Chidester, Tianming Zhou, Shahul Alam and Jian Ma, 9 January 2023, Nature Genetics.
DOI: 10.1038/s41588-022-01256-z
But what if it doesn’t identify as a cell?