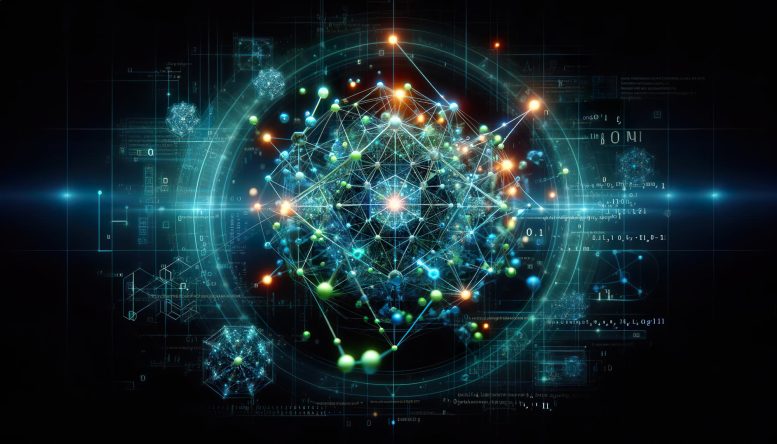
Innovative research utilizing machine learning to analyze atomic pieces of geometry has marked a major advancement in mathematics, potentially revolutionizing the field by speeding up the identification and understanding of complex geometric shapes and patterns.
New research has leveraged machine learning techniques to identify the properties of atomic pieces of geometry. This groundbreaking effort holds the potential to propel the advancement of novel findings in the field of mathematics.
Mathematicians from the University of Nottingham and Imperial College London have, for the first time, used machine learning to expand and accelerate work identifying ‘atomic shapes’ that form the basic pieces of geometry in higher dimensions. Their findings have been published in Nature Communications.
Developing a ‘Periodic Table’ for Geometric Shapes
The research group started their work to create a Periodic Table for shapes several years ago. The atomic pieces are called Fano varieties. The team associates a sequence of numbers, called quantum periods, to each shape, giving a ‘barcode’ or ‘fingerprint’ that describes the shape. Their recent breakthrough uses a new machine learning methodology to sift very quickly through these barcodes, identifying shapes and their properties such as the dimension of each shape.
Insights from the Research Team
Alexander Kasprzyk is an Associate Professor in Geometry in the School of Mathematical Sciences at the University of Nottingham and was one of the authors on the paper. He explains: “For mathematicians, the key step is working out what the pattern is in a given problem. This can be very difficult, and some mathematical theories can take years to discover.”
Professor Tom Coates from the Department of Mathematics at Imperial College London and co-author on the paper said, “This is where Artificial Intelligence could really revolutionize Mathematics as we have shown that machine learning is a powerful tool for spotting patterns in complex domains like algebra and geometry.”
Sara Veneziale, co-author and a PhD student in the team, continues: “We’re really excited about the fact that machine learning can be used in Pure Mathematics. This will accelerate new insights across the field.”
Reference: “Machine learning the dimension of a Fano variety” by Tom Coates, Alexander M. Kasprzyk and Sara Veneziale, 8 September 2023, Nature Communications.
DOI: 10.1038/s41467-023-41157-1
According to the theory of topological vortex gravitational field, the complex geometric shapes and patterns of topological vortices can explain the problem of atomic gravity and be used to construct more new types of particles.
If you are interested, you can browse https://zhuanlan.zhihu.com/p/624024548 or The comments of https://scitechdaily.com/microscope-spacecrafts-most-precise-test-of-key-component-of-the-theory-of-general-relativity/.
Good luck to you all.
I have read some articles about how AI sees a shape and draws it at the same time and the two really don’t have the same picture in thought image communication would this be a problem with this approach or is AI seeing what is described.
According to topological vortex gravitational field theory, observation and measurement are also a form of interaction.
… Did those scientist take in consideration that drip-drop array?