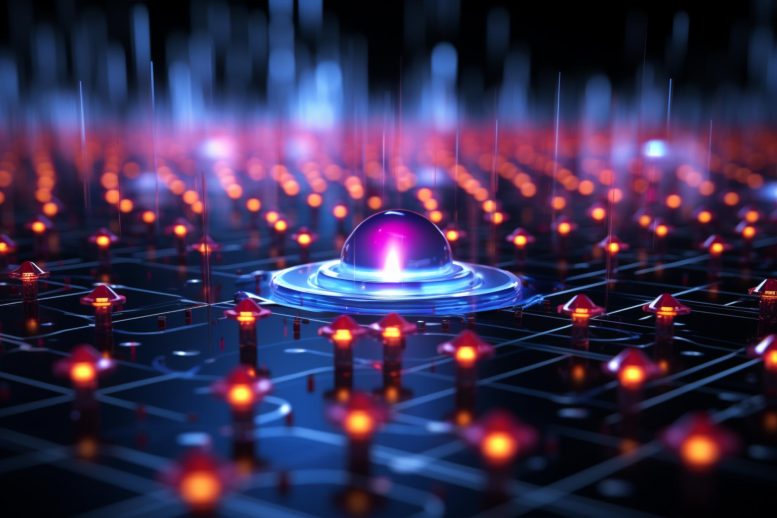
Researchers have made a breakthrough in optical computing by developing a 5-bit photonic memory that allows for fast training and highly energy-efficient processes. The new technology suggests a promising future for optical computing, despite existing challenges.
In a breakthrough for optical computing, researchers developed a nanosecond-scale volatile modulation scheme integrating a phase-change material.
Technological advancements such as autonomous driving and computer vision have spurred a significant increase in demand for computational power. Optical computing, characterized by its high throughput, energy efficiency, and low latency, has attracted significant interest from both academia and industry. However, current optical computing chips are hampered by their power consumption and size, which limit the scalability of optical computing networks.
Nonvolatile integrated photonics has emerged to address these issues, offering optical computing devices the ability to perform in-memory computing while operating with zero static power consumption. Phase-change materials (PCMs), with their high refractive index contrast between different states and reversible transitions, have become promising candidates for enabling photonic memory and nonvolatile neuromorphic photonic chips. This makes PCMs ideally suited for large-scale nonvolatile optical computing chips.
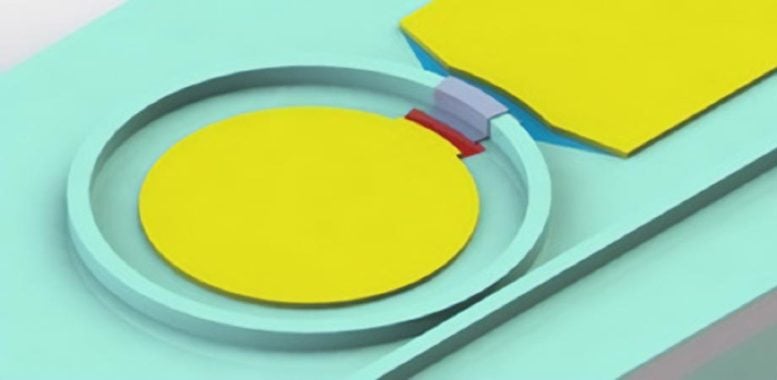
New approach to photonic memory integrates a phase-change material to enable in-situ training of optical neural networks. Credit: Maoliang Wei and Kai Xu
While the potential of nonvolatile integrated optical computing chips is exciting, it comes with its share of challenges. One key hurdle is the requirement for frequent and rapid switching, which is essential for online training. Overcoming this obstacle to ensure quick and efficient training is a critical step in unlocking the full potential of photonic computing chips.
Recently, a significant breakthrough was made by researchers from Zhejiang University, Westlake University, and the Institute of Microelectronics of the Chinese Academy of Sciences. As detailed in the journal Advanced Photonic, they developed a 5-bit photonic memory capable of fast volatile modulation and proposed a solution for a nonvolatile photonic network supporting rapid training. This achievement was made possible by integrating the low-loss PCM antimonite (Sb2S3) into a silicon photonic platform.
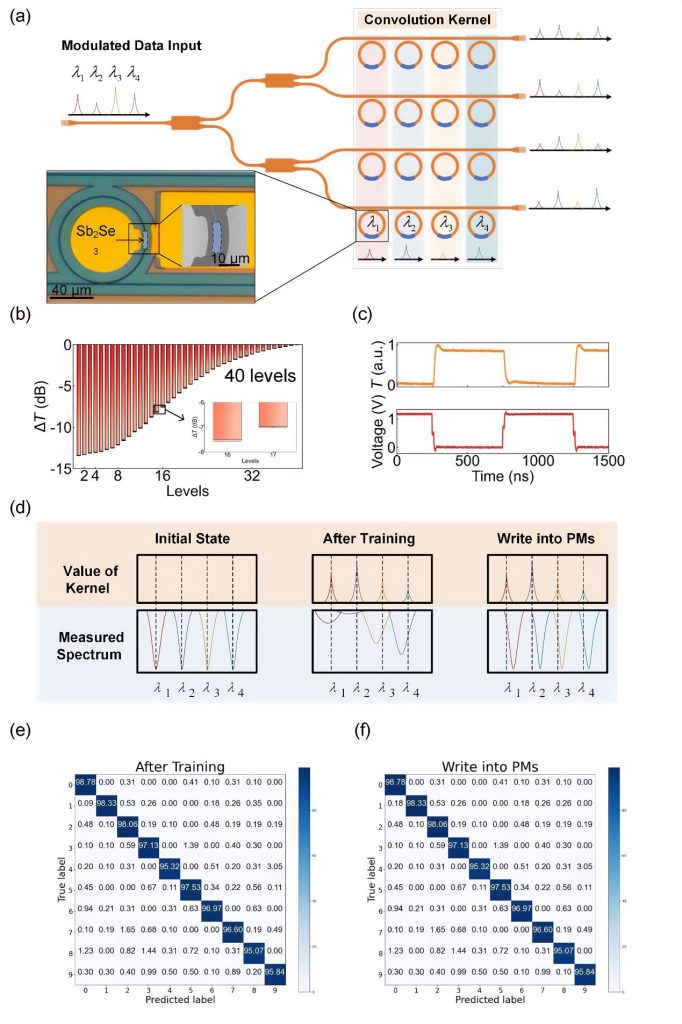
Optical convolution kernel based on the volatile-modulation-compatible photonic memory: (a) Schematic architecture of a 4×4 OCK. The inset is the discrete device of OCK. (b) The nonvolatile multi-level switching of photonic memory. (c) The volatile modulation dynamic response of the photonic memory. (d) Schematic diagram of the on-chip training and writing operation of the OCK. (e) The prediction accuracy after simulated in-situ training of the OCK. (f) The prediction accuracy after the simulated memorizing of photonic memory of the OCK. Credit: M. Wei et al., doi 10.1117/1.AP.5.4.046004
The photonic memory uses the carrier dispersion effect of a PIN diode to achieve volatile modulation with a rapid response time of under 40 nanoseconds, preserving the stored weight information. After training, the photonic memory leverages the PIN diode as a microheater to enable multilevel and reversible phase changes of Sb2S3, allowing the storage of trained weights in the photonic computing network. This leads to an incredibly energy-efficient photonic computing process.
Using the demonstrated photonic memory and its working principle, the research team simulated an optical convolutional kernel architecture. Remarkably, they achieved over 95 percent accuracy in recognizing the MNIST dataset. This showcases the feasibility of fast training through volatile modulation and weight storage through 5-bit nonvolatile modulation.
This groundbreaking research paves the way for a new paradigm in photonic memory and offers a promising solution for the implementation of nonvolatile devices in fast-training optical neural networks. Given these advancements, the future of optical computing looks brighter than ever before.
Reference: “Electrically programmable phase-change photonic memory for optical neural networks with nanoseconds in situ training capability” by Maoliang Wei, Junying Li, Zequn Chen, Bo Tang, Zhiqi Jia, Peng Zhang, Kunhao Lei, Kai Xu, Jianghong Wu, Chuyu Zhong, Hui Ma, Yuting Ye, Jialing Jian, Chunlei Sun, Ruonan Liu, Ying Sun, Wei. E. I. Sha, Xiaoyong Hu, Jianyi Yang, Lan Li and Hongtao Lin, 18 July 2023, Advanced Photonics.
DOI: 10.1117/1.AP.5.4.046004
Be the first to comment on "Unleashing Photonic Power: Groundbreaking Advancements in Optical Computing"